Brain2GAN: Feature-disentangled neural encoding and decoding of visual perception in the primate brain
Thirza Dado, Paolo Papale, Antonio Lozano, Lynn Le, Feng Wang, Marcel van Gerven, Pieter Roelfsema, Yağmur Güçlütürk & Umut Güçlü
PLoS Computational Biology
07 May 2024
📝 Full paper
🔑 GitHub repo
👩🦰 Face stimuli and responses
🌄 Image stimuli and responses
🪧 Poster
Abstract
A challenging goal of neural coding is to characterize the neural representations underlying visual perception. To this end, multi-unit activity (MUA) of macaque visual cortex was recorded in a passive fixation task upon presentation of faces and natural images. We analyzed the relationship between MUA and latent representations of state-of-the-art deep generative models, including the conventional and feature-disentangled representations of generative adversarial networks (GANs) (i.e., z- and w-latents of StyleGAN, respectively) and language-contrastive representations of latent diffusion networks (i.e., CLIP-latents of Stable Diffusion). A mass univariate neural encoding analysis of the latent representations showed that feature-disentangled w representations outperform both z and CLIP representations in explaining neural responses. Further, w-latent features were found to be positioned at the higher end of the complexity gradient which indicates that they capture visual information relevant to high-level neural activity. Subsequently, a multivariate neural decoding analysis of the feature-disentangled representations resulted in state-of-the-art spatiotemporal reconstructions of visual perception. Taken together, our results not only highlight the important role of feature-disentanglement in shaping high-level neural representations underlying visual perception but also serve as an important benchmark for the future of neural coding.
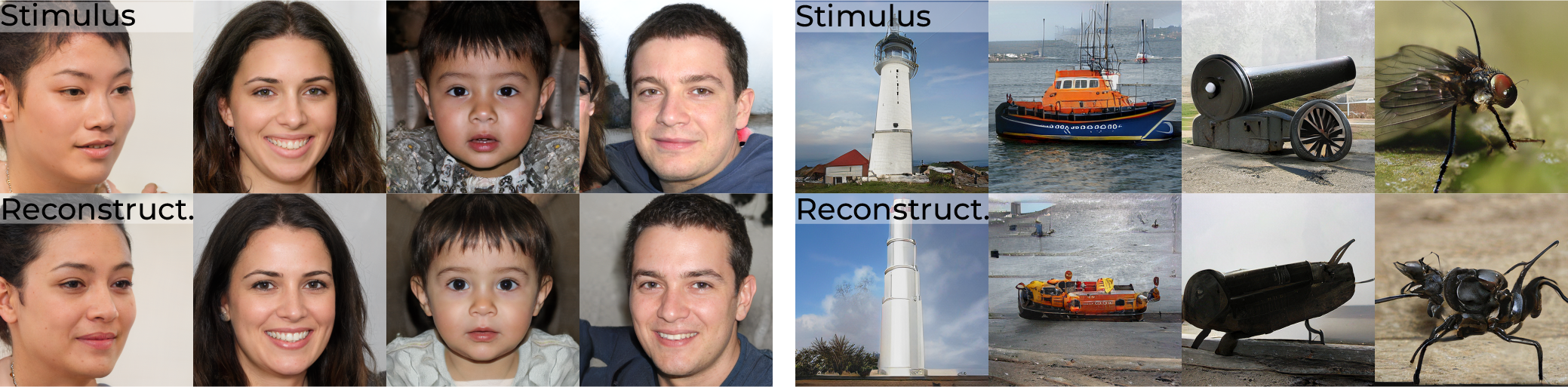
Test set stimulus (top) reconstruction (bottom) from brain activity.